" Carryout an initial PIM filling" or "Take over the product data": Behind these harmless task descriptions, real abysses can open up, which can sometimes bring a PIM or PMDM project into a real imbalance. Let us show you three efficient ways out of product data chaos.
Projects regarding Product Information Management (PIM) - or, more recently, Product Data Master Data Management (PMDM) - consist of many individual work packages and sub-projects. Operational organizations must be created, processes defined and interfaces set up. And as a rule, a PIM-Software which can then be used to manage the new data world conveniently and reliably.
The goal is always to create a new single point of truth (SPOT) for product master data. In other words, a reliable, up-to-date and available database from which all channels can draw. These can be web and print catalogs, major retail platforms, or the company's own e-commerce sales channels, which can then be fed with little effort. But internal customers also benefit, such as product management, customer service or technical documentation.
But how do you get to that single point of truth, especially when that state is miles away from the project? When product information is dispersed in different databases and documents? If there is either outdated and/or unstructured information in the inventory systems? When this whole pile of data just won't fit together?
Let us show you three strategies with which you can organise your product data and build a clean master data basis.
1. A realistic planning
Plan conservatively.
One thing up front: you usually can't expect much help with the upcoming cleanup from the people who set up your PIM or MDM software, the system integrators. There you will usually find excellent developers for customizing these systems, experts for workflows and experienced consultants for data models. But the initial filling itself is mostly left to you. The offers then often speak of "support for data transfer". But support also means exactly that: We show you how to do it, but then you have to do it yourself.
The reason is clear. There is a high effort and cost risk in data transfer. Therefore, much of the actual work will fall on your staff. Or you can seek dedicated external support for this topic - from proven data specialists who are no strangers to AI-supported product data classification, ETL and XSLT transformations.
After all, if the product knowledge is already there but needs to be recombined and consolidated, the data transfer can also be automated to a certain extent. Even then, however, it will not be possible to do it entirely without manual labor, because even an artificial intelligence must first be "trained" before it can make accurate decisions.
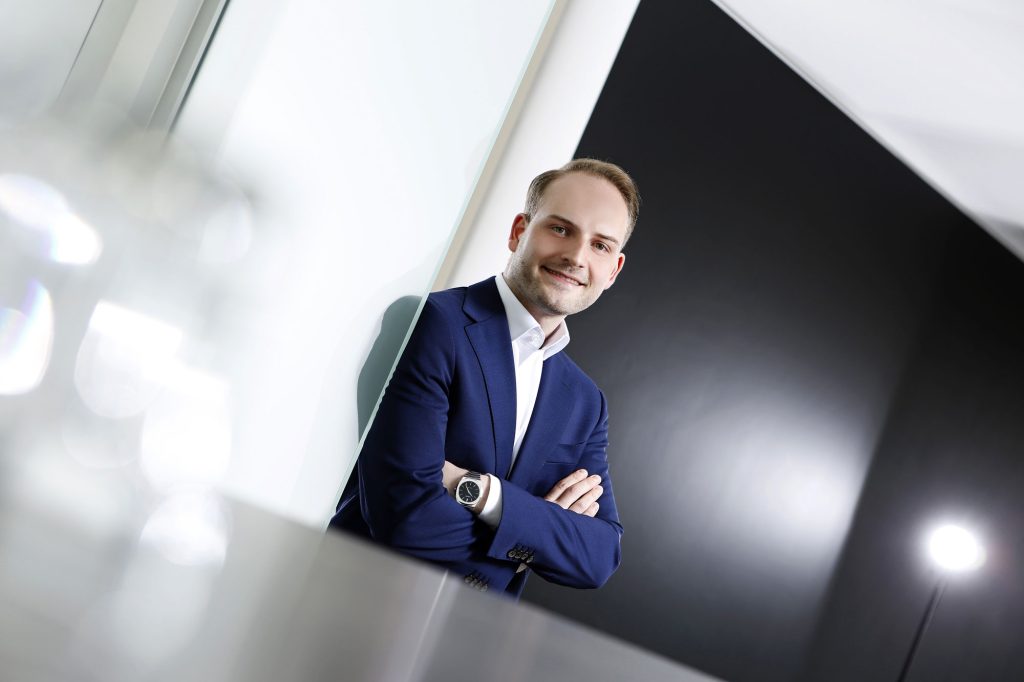
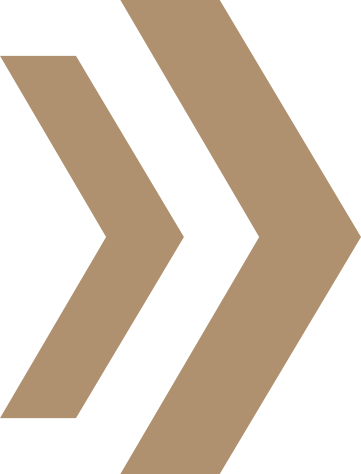
"Our advice: plan enough time and capacity for the data move.
In our experience, 30 to 60 % of the project effort in PIM projects goes into data consolidation and transfer.
So don't underestimate it."
Tarek Nitschke, Product Data Service Expert at Xtentio GmbH
2. A suitable data model for your products
Excellent product data quality starts with the data model.
If your current product data information management consists rather of "grown structures", it is time to think fundamentally about a clean and future-oriented data model in which you want to map your products. You should keep the following in mind:
Create a model that fits your product world.
There is no universal standard for the perfect product data model. Therefore, in PIM systems data structures can be created with a fairly high degree of freedom. However, not every structure is suitable for every product range. For an industrial company with mechatronic products and numerous components and accessories, complex object dependencies play an important role.
For a wholesaler of office equipment, the focus will be on product data classification that is as simple as possible. Therefore, your data model requirements must be clear. Based on these requirements, the integrator can then implement the optimal product data structure for your assortment in the system.
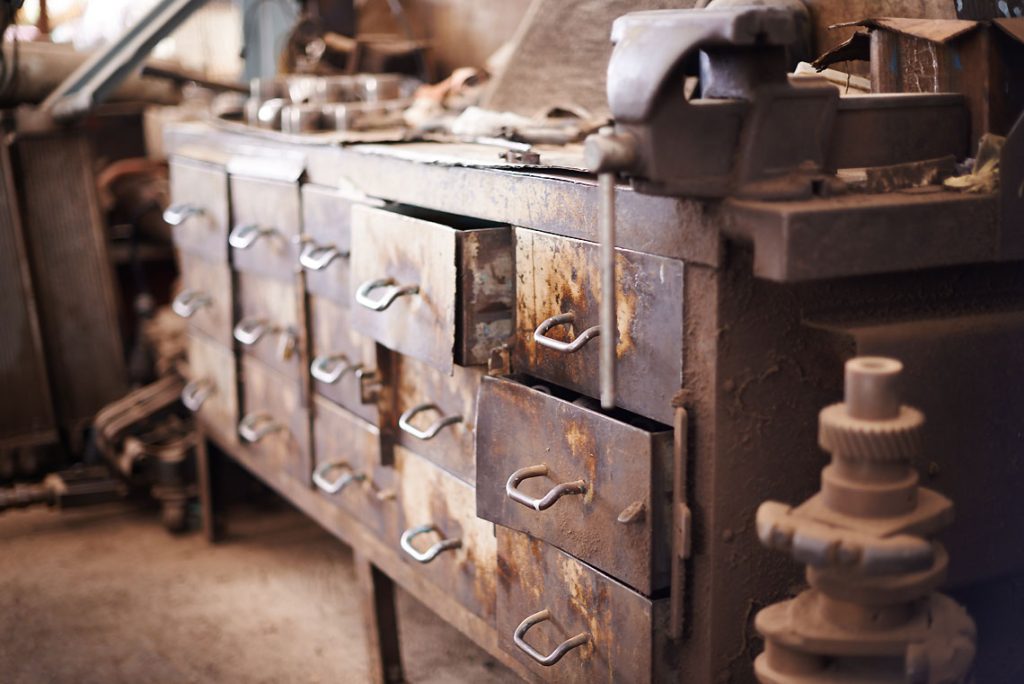
What is a product data model?
A product data model can be thought of as an organization or drawer system for product master data. It is determined in which structures and hierarchy nodes the products are located, which products belong to which classes and which properties, characteristics and relationships characterize the products.
A power cable is sorted in the product tree under the product group "Accessories" in the product class "Connecting cables" and can assume various product properties such as length, diameter, tensile strength or permissible ambient conditions.
Complex products, such as an electric drive, are filed in the "Drives" product group. They belong to a different class, for example, the "electric motors". This class will then also contain considerably more product properties, such as performance specifications, dimensions, mechanical properties, electrical properties, software versions, etc. And probably a relationship between the drive and the matching connection cable will also be possible.
Output classifications for data exchange such as ETIM and eCl@ss will deviate significantly from this "logical" product data model. However, it makes sense to consider the requirements and needs of eCl@ss and Co. when designing the product data model.
Don't have undue respect for your legacy systems.
If you want to turn a data garden that has grown wild over many years into a smart product data warehouse, you will not be able to avoid tearing it down and building a new one. Build a "new world" with product data structures that are as accurate as possible. Make use of all the possibilities and modeling concepts that the new PIM system. Compromises with the "old world" may speed up the data transfer, but they will jeopardize the usability of your product data in the long term. And even SAP and the like cannot usually contribute much to the new product data structures - but be sure to consider the requirements for unique identifiers such as SAP material numbers in the data model.
Don't think in terms of output or catalog structures.
It is often argued that with eCl@ss, ETIM, BMEcat or other classification standards, everything is already predefined. It would therefore suffice to map these structures in PIM and that would be the end of the matter. A clear no! These are standards for data exchange that are not suitable for mapping your product world in all its facets and relationships. Don't worry: almost all PIM systems provide output modules with which you can later generate print catalogs, standardized exchange formats or special exports, for example for certain wholesalers. Or you can use special data mapping tools for this task, such as Productsup or Talend.
3. Data consolidation before launch
The big cleanup before data transfer.
When you move house, you do the same thing: a few weeks beforehand, you place classified ads or order bulky waste, get rid of all the superfluous ballast, and only take what you really need with you to your new home. Exactly this strategy is also recommended for the initial filling of your new PIM or PMDM system.
Clean up properly beforehand and consolidate your product data. Don't be afraid to discard incorrect, unstructured, incomplete and outdated data if it doesn't add value.
Discard redundant data
If you need to migrate data from different source systems, check which information is redundant and which is complementary. Discard data sources that do not contribute significantly to the new SPOT.
Classify cleanly
If you haven't had a clean product data classification until now, it's very likely that you'll need to sort your products into these new pigeonholes. For less than 10,000 products, this can still be done manually, for example with the help of Excel. When it becomes more, AI tools that use similarity analysis to pre-sort help.
Atomize data
It also happens that you need to "atomize" data. This is because descriptive texts - such as "The operating temperature of the device is between -30 and 70 °C at a maximum relative humidity of 80 %" - unfortunately cannot do anything with a facet search. In this case, too, AI tools can use pattern recognition to help automate the breakdown into individual features.
Sort out historical data
Check which data is up to date. You will only take historical data with you into the new system in absolutely exceptional cases.
Standardize terminology
After that, it's the turn of the values and designations. It becomes difficult when the same things or properties are described differently. This can happen if no clear terminology is defined. Sometimes the housing material is referred to as "stainless steel", "1.4301" or "V2A" - but all these terms mean the same thing. For a clean single point of truth, values and product names must be standardized, preferably in a form that is most familiar to your customers.
Consider country-specific information
Also, don't forget about the different languages and country-specific data. A text that is available in 24 languages must also be considered, transformed, correctly sorted and quality-assured 24 times during initial filling.
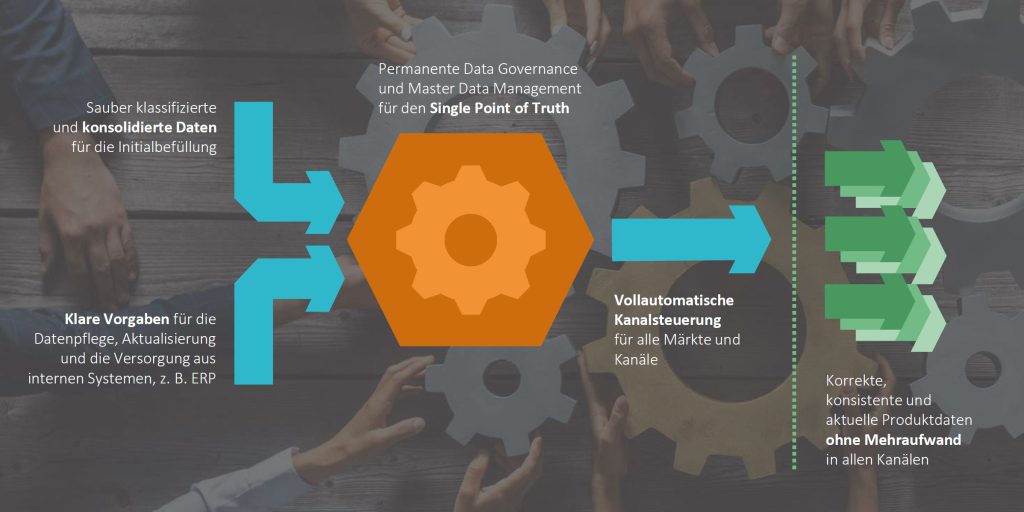
These are just a few examples of the extensive data consolidationyou should perform before moving to your new single point of truth. Not all activities are always necessary, but in our experience, the effort behind them is chronically underestimated. This is not helped by temporary staff who simply transfer data from the legacy systems to the new product data world. A manual transfer - without having the necessary expertise - usually leads to errors that then have to be laboriously corrected again afterwards.
And it is worth it after all
Every initial filling is completed at some point, the data is consolidated, transferred to the new PIM system. The data has been transferred, finally quality-assured and is available in the new system in the best quality, structure and usability. An important milestone has been reached: you have created a clean "version 1.0" of your product master data, the single point of truth with which you can supply all channels in future. This results in numerous advantages.
Customer Experience: High-quality product data ensures a consistent approach to your customers across different sales and marketing channels. They enable comprehensive filtering, search and comparison options in web catalogs and web stores.
Scalability: Roll-outs of a wide variety of new marketing and sales channels are simplified by connecting and activating them once. This means that many channels can be operated fully automatically and without manual effort.
Automation:Consistent and uniformly structured product data enables the automation of many processes, from evaluation and analysis to the rule-based derivation of data into the various trading platforms.
Internationalization: Centrally organized and structured product data enables uniform translation and market-specific adaptation of product information in order to quickly expand business activities to additional countries and markets.
More sales in the long term: Comparable data, clean classifications, search engine optimized content and appealing product images help you stand out from the competition and promote trust in your products.
Cost reduction: Manual data management tasks are eliminated, returns are avoided, and costly data corrections are a thing of the past: High-quality data sustainably reduces direct and indirect operating costs.
So the big cleanup is worth it. In the next part, you'll learn how your product master data stays in shape for the long term once you're up and running. There we report on how you can maintain and improve your product data quality in the long term with Data Governance and simple rules.
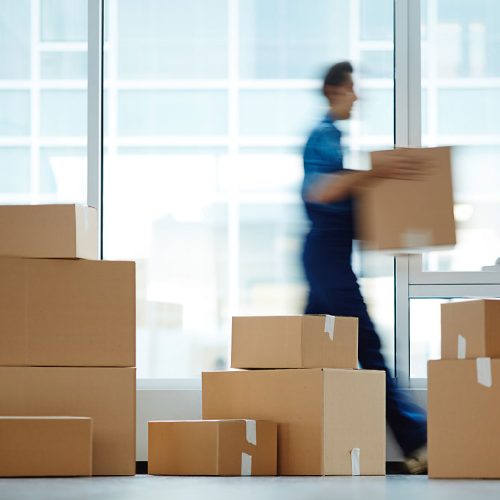
We make your data ready to move!
In order not to jeopardize the success of your digitization projects and to resolve short-term resource bottlenecks, we support you flexibly and professionally with our product data services. Learn how to make your product data fit for new applications and opportunities!
Are you interested in this topic?
Read more: möbelfertigung. Bundling data management at a central location.
Find more information: Software selection and rollout of global PIM and DAM systems at Beiersdorf AG
or
Get in touch with us to orchestrate your individual situation. We will be happy to share exciting insights into industry-specific features and best practices and provide you with individual recommendations for your company.